Methodology#
For this scoping review, we focused on research articles published in the journal Magnetic Resonance in Medicine (MRM). In addition to being a journal primarily dedicated to the development of MRI techniques, MRM is also at the forefront of promoting reproducible research practices. Since 2020, the journal has singled out 31 research articles that promote reproducibility in MRI, and has published a series of interviews with the article authors, discussing the tools and practicesapproaches they used to bolster the reproducibility of their findings. These interviews are freely available on the MRM Highlights portal under the label ‘reproducible research insights’.
To see how these articles relate to other literature dedicated to reproducibility in MRI, we conducted a literature search utilizing the Semantic Scholar API [Fricke, 2018] with the following query terms: (code | data | open-source | github | jupyter ) & ((MRI & brain) | (MRI & neuroimaging)) & reproducib~
. This search ensured that the scoping review is informed by an a priori literature search protocol that is transparent and reproducible, presenting the data in a structured way.
Show code cell source
import requests
from thefuzz import fuzz
import json
import time
import numpy as np
import umap
import os
import pandas as pd
import umap.plot
import plotly.graph_objects as go
from plotly.offline import plot
from IPython.display import display, HTML
import base64
# REQUIRED CELL
DATA_ROOT = "../data/repro_mri_scoping"
def get_by_id(paper_id):
response = requests.post(
'https://api.semanticscholar.org/graph/v1/paper/batch',
params={'fields': 'abstract,tldr,year,embedding'},
json={"ids": [paper_id]})
if response.status_code == 200:
return response
else:
return None
def get_id(title):
"""
Query Semantic Scholar API by title.
"""
api_url = "https://api.semanticscholar.org/graph/v1/paper/search"
params = {"query": title}
response = requests.get(api_url, params=params)
if response.status_code == 200:
result = response.json()
print(result['total'])
for re in result['data']:
print(re)
if fuzz.ratio(re['title'],title) > 90:
return re['paperId']
else:
return None
else:
return None
def bulk_search(query,save_json):
"""
The returns 1000 results per query. If the total number of
hits is larger, the request should be iterated using tokens.
"""
query = "(code | data | open-source | github | jupyter ) + (('MRI' + 'brain') | (MRI + 'neuroimaging')) + reproducib~"
fields = "abstract"
url = f"http://api.semanticscholar.org/graph/v1/paper/search/bulk?query={query}&fields={fields}"
r = requests.get(url).json()
print(f"Found {r['total']} documents")
retrieved = 0
with open(save_json, "a") as file:
while True:
if "data" in r:
retrieved += len(r["data"])
print(f"Retrieved {retrieved} papers...")
for paper in r["data"]:
print(json.dumps(paper), file=file)
if "token" not in r:
break
r = requests.get(f"{url}&token={r['token']}").json()
print(f"Retrieved {retrieved} papers. DONE")
def read_json_file(file_name):
with open(file_name, 'r') as json_file:
json_list = list(json_file)
return json_list
def write_json_file(file_name, dict_content):
with open(file_name, 'w') as json_file:
json_file.write(json.dumps(dict_content))
def get_output_dir(file_name):
op_dir = "../output"
if not os.path.exists(op_dir):
os.mkdir(op_dir)
return os.path.join(op_dir,file_name)
def flatten_dict(input):
result_dict = {}
# Iterate over the list of dictionaries
for cur_dict in input:
# Iterate over key-value pairs in each dictionary
for key, value in cur_dict.items():
# If the key is not in the result dictionary, create a new list
if key not in result_dict:
result_dict[key] = []
# Append the value to the list for the current key
result_dict[key].append(value)
return result_dict
/Users/agah/opt/anaconda3/envs/t1book/lib/python3.8/site-packages/umap/plot.py:203: NumbaDeprecationWarning: The keyword argument 'nopython=False' was supplied. From Numba 0.59.0 the default is being changed to True and use of 'nopython=False' will raise a warning as the argument will have no effect. See https://numba.readthedocs.io/en/stable/reference/deprecation.html#deprecation-of-object-mode-fall-back-behaviour-when-using-jit for details.
@numba.jit(nopython=False)
Show code cell source
#OPTIONAL CELL
literature_records = get_output_dir("literature_records.json")
search_terms = "(code | data | open-source | github | jupyter ) + (('MRI' + 'brain') | (MRI + 'neuroimaging')) + reproducib~"
# This will save output/literature_records.json
bulk_search(search_terms,literature_records)
Found 1080 documents
Retrieved 999 papers...
Retrieved 1079 papers...
Retrieved 1079 papers. DONE
Add articles associated with the reproducibility insights#
Among 1098 articles included in the these Semantic Scholar records, SPECTER vector embeddings [Cohan et al., 2020] were available for 612 articles, representing the publicly accessible content in abstracts and titles. The high-dimensional semantic information captured by the word embeddings was visualized using the uniform manifold approximation and projection method [McInnes et al., 2018].
Crawling content
To include the paper IDs of the articles highlighted by reproducibility insights, we will utilize filenames from the repro_mri_scoping/repro_insights_parsed_nov23
directory. For more information about this dataset, please refer to the following details.
In order to reduce crawler activity on the target website, spider commands are not included in the notebook. Instead, the content obtained by using the crawler (November 2023) was provided as part of the dataset (repro_mri_scoping/repro_insights_parsed_nov23
).
You can run the following commands in a terminal to re-run the crawler:
cd crawler
scrapy crawl mrmh
This will save the outputs in crawler/crawler_outputs
directory. Files are named according to the captured URLs. To expand or narrow down the search interval, please modify the following block in crawler/insights/spiders/mrmh.py
:
for year in range(0, 4) # assuming 2023
for month in range(1, 13)
for day in range(1, 32)]
After the crawling process is completed, the details of the article upon which the interview is based will be retrieved from the Semantic Scholar API. Following this, title
, abstract
, and TLDR
(if availabe) sections will be prepended to each document. To perform these tasks on the crawled content, please run the following in terminal:
python parse_crawled_content.py
Parsed outputs will be saved to crawler/crawler_output/collection
, filenames will attain paperId
(the uniqe ID assigned by Semantic Scholar).
Note
Some of the content fetched from the web needs minor edits. Please see crawler/insights/spiders/NOTES.txt
.
Show code cell source
# REQUIRED CELL
# To load THE ORIGINAL LIST, please comment in the following
lit_list = read_json_file(os.path.join(DATA_ROOT,"literature_records.json"))
# Read the LATEST literature records returned by the above search
# Note that this may include new results (i.e., new articles)
#literature_records = get_output_dir("literature_records.json")
#lit_list = read_json_file(literature_records)
# Collect all the paper IDs from the literature search
lit_ids = [json.loads(entry)['paperId'] for entry in lit_list]
# Get all paper ids for the articles linked to
insights_path = os.path.join(DATA_ROOT,"repro_insights_parsed_nov23")
insights_ids = [f.split(".")[0] for f in os.listdir(insights_path) if f.endswith('.txt')]
# Combine all IDs (unique)
paper_ids_all = list(set(lit_ids + insights_ids))
print(f"Total: {len(paper_ids_all)} papers ")
Total: 1098 papers
Note
The following cell is commented out as it involves a series of API calls that takes some time to complete.
Show code cell source
# OPTIONAL CELL
# slices = [(0, 499), (499, 998), (998, None)]
# request_fields = 'title,venue,year,embedding,citationCount'
# results = []
# for start, end in slices:
# print(len(paper_ids_all[start:end]))
# re = requests.post(
# 'https://api.semanticscholar.org/graph/v1/paper/batch',
# params={'fields': request_fields},
# json={"ids": paper_ids_all[start:end]})
# if re.status_code == 200:
# print(f"Got results {start}:{end} interval")
# results.append(re.json())
# time.sleep(15) # Rate limiting.
# else:
# print(f"WARNING slice {start}:{end} did not return results: {re.text}")
# ALTERNATIVE
# The above API call should work fast as the requests are sent in batch.
# However, it frequently throws 429 error. If that's the case, following will
# also work, but takes much longer and a few articles may not be captured.
# results = []
# for cur_id in paper_ids_all:
# #print(len(paper_ids_all[start:end]))
# re = requests.get(
# f'https://api.semanticscholar.org/graph/v1/paper/{cur_id}',
# params={'fields': request_fields})
# if re.status_code == 200:
# results.append(re.json())
# else:
# print(f"WARNING request for {cur_id} could not return results: {re.text}")
# # Write outputs
# write_json_file(get_output_dir("literature_data.json"),results)
Show code cell source
# REQUIRED CELL
# Load the ORIGINAL data
lit_data = json.loads(read_json_file(os.path.join(DATA_ROOT,"literature_data.json"))[0])
# If you'd like to read from the output directory (LATEST)
#lit_data = json.loads(read_json_file(get_output_dir("literature_data.json"))[0])
papers_data = []
for res in lit_data:
if 'embedding' in res.keys():
if res['embedding']:
cur_rec = {"embedding":res['embedding']['vector'],
"title":res['title'],
"venue": res['venue'],
"year": res['year'],
"is_mrmh": "Other",
"paperId": res['paperId'],
"n_citation": res['citationCount']}
if res['paperId'] in insights_ids:
cur_rec['is_mrmh'] = "Highlights"
papers_data.append(cur_rec)
papers_data_dict = papers_data
# From a list of dicts to a dict of lists.
papers_data = flatten_dict(papers_data)
Dimension reduction and visualization
To visualize the semantic relationship between the captured articles (a 612x768 matrix), we are going to use Uniform Manifold Approximation and Projection (UMAP) method.
Show code cell source
# REQUIRED CELL
# Reduce to 2D feature
umap_model_2d = umap.UMAP(n_neighbors=15, min_dist=0.1, n_components=2,random_state=42)
umap_2d = umap_model_2d.fit_transform(np.array(papers_data['embedding']))
umap_2d_mapper = umap_model_2d.fit(np.array(papers_data['embedding']))
# Reduce to 3D feature
umap_model_3d = umap.UMAP(n_neighbors=15, min_dist=0.1, n_components=3,random_state=42)
umap_3d = umap_model_3d.fit_transform(np.array(papers_data['embedding']))
/Users/agah/opt/anaconda3/envs/t1book/lib/python3.8/site-packages/umap/umap_.py:1943: UserWarning:
n_jobs value -1 overridden to 1 by setting random_state. Use no seed for parallelism.
/Users/agah/opt/anaconda3/envs/t1book/lib/python3.8/site-packages/numba/np/ufunc/parallel.py:371: NumbaWarning:
The TBB threading layer requires TBB version 2021 update 6 or later i.e., TBB_INTERFACE_VERSION >= 12060. Found TBB_INTERFACE_VERSION = 12030. The TBB threading layer is disabled.
OMP: Info #273: omp_set_nested routine deprecated, please use omp_set_max_active_levels instead.
/Users/agah/opt/anaconda3/envs/t1book/lib/python3.8/site-packages/umap/umap_.py:1943: UserWarning:
n_jobs value -1 overridden to 1 by setting random_state. Use no seed for parallelism.
/Users/agah/opt/anaconda3/envs/t1book/lib/python3.8/site-packages/umap/umap_.py:1943: UserWarning:
n_jobs value -1 overridden to 1 by setting random_state. Use no seed for parallelism.
Show code cell source
umap.plot.connectivity(umap_2d_mapper, edge_bundling='hammer')
/Users/agah/opt/anaconda3/envs/t1book/lib/python3.8/site-packages/umap/plot.py:894: UserWarning:
Hammer edge bundling is expensive for large graphs!
This may take a long time to compute!
<Axes: >
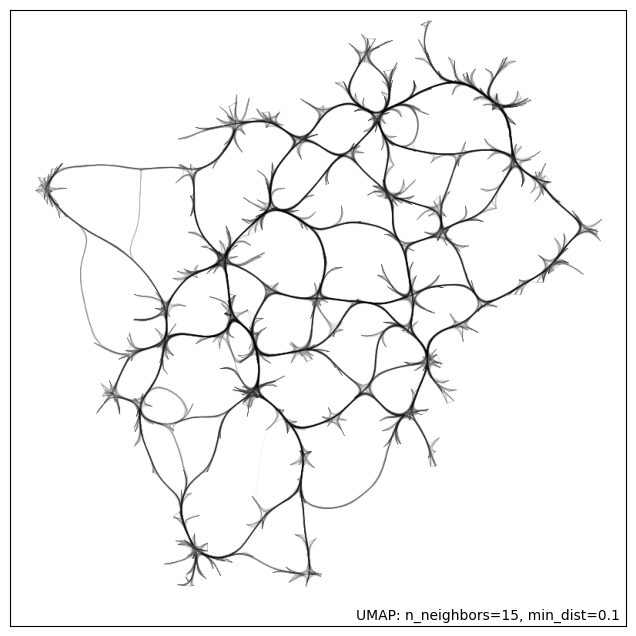
The MRI systems cluster was predominantly composed of articles published in MRM, with only two publications appearing in a different journal [Adebimpe et al., 2022, Tilea et al., 2009]. Additionally, this cluster was sufficiently distinct from the rest of the reproducibility literature, as can be seen by the location of the dark red dots on Fig. 1.
Show code cell source
# Create Plotly figure
fig = go.Figure()
# Scatter plot for UMAP in 2D
scatter_2d = go.Scatter(
x=umap_2d[:, 0],
y=umap_2d[:, 1],
mode='markers',
marker = dict(color =["#562135" if item == 'Highlights' else "#f8aabe" for item in papers_data['is_mrmh']],
size=9,
line= dict(color="#ff8080",width=1),
opacity=0.9),
customdata= [f"<b>{dat['title']}</b> <br>{dat['venue']} <br>Cited by: {dat['n_citation']} <br>{dat['year']}" for dat in papers_data_dict],
hovertemplate='%{customdata}',
visible = True,
name='2D'
)
fig.add_trace(scatter_2d)
# Add dropdown
fig.update_layout(
updatemenus=[
dict(
type = "buttons",
direction = "left",
buttons=list([
dict(
args=[{"showscale":True,"marker": dict(color =["#562135" if item == 'Highlights' else "#f8aabe" for item in papers_data['is_mrmh']],
size=9,
line= dict(color="#ff8080",width=1),
opacity=0.9)}],
label="Highlights",
method="restyle"
),
dict(
args=[{"marker": dict(color = np.log(papers_data['n_citation']),colorscale='Plotly3',size=9, colorbar=dict(thickness=10,title = "Citation (log)",tickvals= [0,max(papers_data['n_citation'])]))}],
label="Citation",
method="restyle"
),
dict(
args=[{"marker": dict(color = papers_data['year'],colorscale='Viridis',size=9,colorbar=dict(thickness=10, title="Year"))}],
label="Year",
method="restyle"
)
]),
pad={"r": 10, "t": 10},
showactive=True,
x=0.11,
xanchor="left",
y=0.98,
yanchor="top"
),
]
)
plotly_logo = base64.b64encode(open(os.path.join(DATA_ROOT,'sakurabg.png'), 'rb').read())
fig.update_layout(plot_bgcolor='white',
images= [dict(
source='data:image/png;base64,{}'.format(plotly_logo.decode()),
xref="paper", yref="paper",
x=0.033,
y=0.956,
sizex=0.943, sizey=1,
xanchor="left",
yanchor="top",
#sizing="stretch",
layer="below")])
fig.update_layout(yaxis={'visible': False, 'showticklabels': False})
fig.update_layout(xaxis={'visible': False, 'showticklabels': False})
# Update layout
fig.update_layout(title='Sentient Array of Knowledge Unraveling and Assessment (SAKURA)',
height = 850,
width = 884,
hovermode='closest')
plot(fig, filename = 'sakura.html')
display(HTML('sakura.html'))
/var/folders/wf/n68nr3yx7pb2yldhq8nrfmzr0000gn/T/ipykernel_280/4278698386.py:36: RuntimeWarning:
divide by zero encountered in log
Figure-1: Edge-bundled connectivity of the 612 articles identified by the literature search. A notable cluster (red) is formed by the MRM articles that were featured in the reproducible research insights (purple nodes), particularly in the development of MRI methods. Notable clusters for other studies (pink) are annotated by gray circles.
Show code cell source
fig = go.Figure()
# Scatter plot for UMAP in 3D
scatter_3d = go.Scatter3d(
x=umap_3d[:, 0],
y=umap_3d[:, 1],
z=umap_3d[:, 2],
mode='markers',
marker = dict(color =["#562135" if item == 'Highlights' else "#f8aabe" for item in papers_data['is_mrmh']],
size=9,
line= dict(color="#ff8080",width=1),
opacity=0.9),
customdata= [f"<b>{dat['title']}</b> <br>{dat['venue']} <br>Cited by: {dat['n_citation']} <br>{dat['year']}" for dat in papers_data_dict],
hovertemplate='%{customdata}',
visible = True,
name='3D'
)
fig.add_trace(scatter_3d)
fig.update_layout(
updatemenus=[
dict(
type = "buttons",
direction = "left",
buttons=list([
dict(
args=[{"marker": dict(color =["#562135" if item == 'Highlights' else "#f8aabe" for item in papers_data['is_mrmh']],
size=9,
line= dict(color="#ff8080",width=1),
opacity=0.9)}],
label="Highlights",
method="restyle"
),
dict(
args=[{"marker": dict(color = np.log(papers_data['n_citation']),colorscale='Plotly3',size=9,colorbar=dict(thickness=10,tickvals= [0,max(papers_data['n_citation'])],title="Citation"))}],
label="Citation",
method="restyle"
),
dict(
args=[{"marker": dict(color = papers_data['year'],colorscale='Viridis',size=9,colorbar=dict(thickness=10, title="Year"))}],
label="Year",
method="restyle"
)
]),
pad={"r": 10, "t": 10},
showactive=True,
x=0.11,
xanchor="left",
y=0.98,
yanchor="top"
),
]
)
# Update layout
fig.update_layout(title='UMAP 3D',
height = 900,
width = 900,
hovermode='closest',
template='plotly_dark')
plot(fig, filename = 'sakura3d.html')
display(HTML('sakura3d.html'))
/var/folders/wf/n68nr3yx7pb2yldhq8nrfmzr0000gn/T/ipykernel_280/3321562894.py:36: RuntimeWarning:
divide by zero encountered in log
- 1
Azeez Adebimpe, Maxwell Bertolero, Sudipto Dolui, Matthew Cieslak, Kristin Murtha, Erica B Baller, Bradley Boeve, Adam Boxer, Ellyn R Butler, Phil Cook, Stan Colcombe, Sydney Covitz, Christos Davatzikos, Diego G Davila, Mark A Elliott, Matthew W Flounders, Alexandre R Franco, Raquel E Gur, Ruben C Gur, Basma Jaber, Corey McMillian, ALLFTD Consortium, Michael Milham, Henk J M M Mutsaerts, Desmond J Oathes, Christopher A Olm, Jeffrey S Phillips, Will Tackett, David R Roalf, Howard Rosen, Tinashe M Tapera, M Dylan Tisdall, Dale Zhou, Oscar Esteban, Russell A Poldrack, John A Detre, and Theodore D Satterthwaite. ASLPrep: a platform for processing of arterial spin labeled MRI and quantification of regional brain perfusion. Nat. Methods, 19(6):683–686, June 2022.
- 2
Hilary Arksey and Lisa O'Malley. Scoping studies: towards a methodological framework. Int. J. Soc. Res. Methodol., 8(1):19–32, February 2005.
- 3
William T Clarke, Charlotte J Stagg, and Saad Jbabdi. FSL-MRS: an end-to-end spectroscopy analysis package. Magn. Reson. Med., 85(6):2950–2964, June 2021.
- 4
missing journal in Cohan2020-tw
- 5
Suzanne Fricke. Semantic scholar. J. Med. Libr. Assoc., 106(1):145, January 2018.
- 6
Danielle Levac, Heather Colquhoun, and Kelly K O'Brien. Scoping studies: advancing the methodology. Implement. Sci., 5:69, September 2010.
- 7
missing journal in McInnes2018-sc
- 8
Micah D J Peters, Christina M Godfrey, Hanan Khalil, Patricia McInerney, Deborah Parker, and Cassia Baldini Soares. Guidance for conducting systematic scoping reviews. Int. J. Evid. Based Healthc., 13(3):141–146, September 2015.
- 9
Layla Tabea Riemann, Christoph Stefan Aigner, Ralf Mekle, Oliver Speck, Georg Rose, Bernd Ittermann, Sebastian Schmitter, and Ariane Fillmer. Fourier-based decomposition for simultaneous 2-voxel MRS acquisition with 2SPECIAL. Magn. Reson. Med., 88(5):1978–1993, November 2022.
- 10
Julien Songeon, Sébastien Courvoisier, Lijing Xin, Thomas Agius, Oscar Dabrowski, Alban Longchamp, François Lazeyras, and Antoine Klauser. In vivo magnetic resonance 31 P-Spectral analysis with neural networks: 31P-SPAWNN. Magn. Reson. Med., 89(1):40–53, January 2023.
- 11
Nikola Stikov, Joshua D Trzasko, and Matt A Bernstein. Reproducibility and the future of MRI research. Magn. Reson. Med., 82(6):1981–1983, December 2019.
- 12
B Tilea, C Alberti, C Adamsbaum, P Armoogum, J F Oury, D Cabrol, G Sebag, G Kalifa, and C Garel. Cerebral biometry in fetal magnetic resonance imaging: new reference data. Ultrasound Obstet. Gynecol., 33(2):173–181, February 2009.